
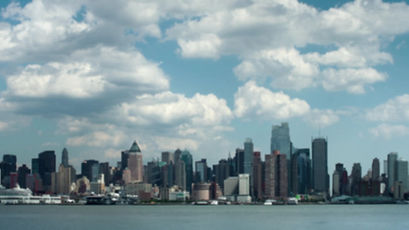
The prevailing characteristics of micro-videos result in the less descriptive power of each modality. The micro-video representations, several pioneer efforts proposed, are limited in implicitly exploring the consistency between different modality information but ignore the complementarity. In this paper, we focus on how to explicitly separate the consistent features and the complementary features from the mixed information and harness their combination to improve the expressiveness of each modality. Towards this end, we present a Neural Multimodal Cooperative Learning model (NMCL) to split the consistent component and the complementary component by a novel relation-aware attention mechanism. Specifically, the computed attention score can be used to measure the correlation between the features extracted from different modalities. And then, a threshold is learned for each modality to distinguish the consistent and complementary features, according to the score. Thereafter, we integrate the consistent parts to enhance the representations and supplement the complementary ones to reinforce the information in each modality. As to the problem of redundant information, which may cause overfitting and is hard to distinguish, we devise an attention network to dynamically capture the features which closely related the category and output a discriminative representation for prediction. Experimental results on a real-world micro-video dataset show that NMCL outperforms state-of-the-art methods. Further studies verify the effectiveness and cooperative effects brought by the attentive mechanism.
An illustraction of our framework. It separates the consistent features from the complementary ones and enhances the expressiveness of each modality via the proposed cooperative net. Then, it selects the features to generate a discriminative representation in the attention network towards venue category estimation.
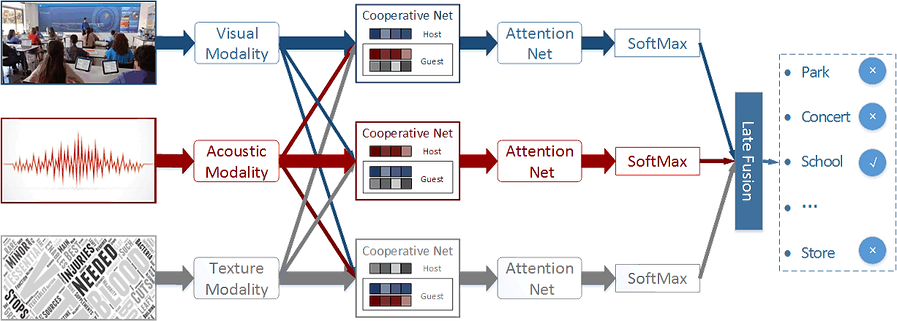
An illustration of our framework. It separates the consistent features from the complementary ones and enhances the expressiveness of each modality via the proposed cooperative net. Then, it selects the features to generate a discriminative representation in the attention network towards venue category estimation.
With the user set, we then crawled the published videos, descriptions and venue information. We crawled the micro-videos from Vine through its public AP. In particular, we first manually chose a small set of active users as the seeds. We expanded the user sets through incrementally gathering the seed users' followers. if available from the collected users. We picked out about 24,000 micro-videos containing Foursquare check-in information from the overall crawled micro-video set. After removing the duplicated venue IDs, we further expanded our video set by crawling all videos in each venue ID with the help of API. Thereafter, we obtained a dataset of 276,264 videos distributed in 442 Foursquare venue categories and served the corresponding ID as the ground truth. Furthermore, we observed that the category distribution is heavily unbalanced. Thereinto, several categories contain only a small number of micro-videos, where it is hard to train a robust classifier. We hence removed the leaf categories with less than 50 micro-videos. At last, we achieved 270,145 micro-videos distributed in 188 Foursquare venue categories.
The code can be available:
The dataset can be available:
-
Textual description (description.text)
-
Raw Feature+Lablel (dataset.h5)
-
Multimodal Features (visual (2048-dim)+acoustic (200-dim)+textual (100-dim))
-
AudioSet